How a leading rebar manufacturer used Digitalization and Industry 4.0 Technologies to implement Material Traceability and Product Genealogy solution
Overview
This case study is of a leading Rebar manufacturers in secondary steel industry well known for its related product brand. It was looking for a solution that will help them digitalize the process of converting their raw material into finished product from the perspective of having traceability of material progressing through different stages in the manufacturing process along with ability to easily retrieve the genealogy of the finished product as and when needed.
The manufacturer reached out to Vega Innovations and Technoconsultants Pvt. Ltd. (VITPL) to help them design and implement the solution. VITPL is a long-term partner of the manufacturer for all their automation needs. For the first phase, VITPL designed the solution by leveraging few of the Industry 4.0 technologies – Sensors, IIoT, WiFi, Internet, and Cloud. The second phase of the solution is targeted to use few additional technologies of Industry 4.0 – Big Data, Analytics and Machine Learning.
Special design and implementation considerations had to be deployed due to the very harsh nature of the manufacturing environment which is typical in steel making industry. For example, extremely high temperature scenarios near furnaces and on the surface of the ladle required special protection designs to avoid burnouts of the sensors and IIoT devices. Different IIoT devices communicated within the plant premises using WiFi.
The software consisted of a core application hosted on cloud with few small modules deployed on-premises for some localised interaction with legacy machines and equipment for data collection.
Approach
The solution divided the manufacturing process, from the point of digitalization, into five areas.
- Melting
- Ladle tracking
- Casting
- Rolling
- Bundling
Melting
The solution involved interfacing with PLCs supporting the furnace operations and deploying additional sensors and IIoT devices to identify start of the heat, end of heat, slag-off events, and tapping events. It also involved creating traceable identifications for heat samples, linking spectrometer testing results to the samples and to the heat.
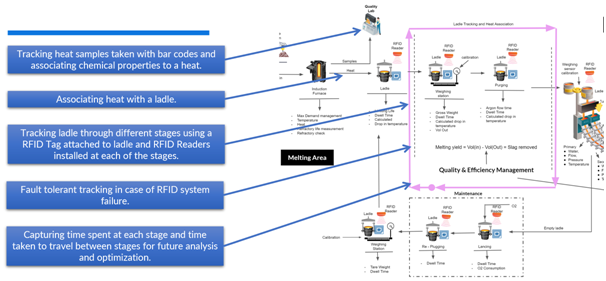
Ladle Tracking
Ladle tracking helps in
- Identifying the heat’s journey accurately from furnace to caster
- Acquiring accurate timestamps for ladle arrivals and departures and accurate transit times, which are useful for crane logistics analysis and planning in future
- Calculating heat losses for waiting time, and correct temperatures for tapping and heating
- Assigning steel weight, remaining weight and tare weight to the ladles, which is important for mass analysis
Passive RFID tags are fixed on ladles using a specially designed RFID Protection Armour. RFID Sensors housed in its own protection housing, leveraged these RFID tags to track position of the ladles. Due to the tags, each ladle gets a unique Identification number. The RFID sensors in furnace area are housed in a specially designed protective cover build using a special material. Even with the protection armour, the RFID tags could be damaged by direct splashes, collision hooks of crane, etc. Thus, the protection armour is designed for fast and simple exchange of the protection armour containing tag. New tags are automatically recognized by the software and the complete process of changing and detecting a new tag is quick. IIoT in other areas were deployed as required. For example, at the weighing station where the ladle is weighed before going to furnace for tapping and after the molten metal is tapped into the ladle is one such position in the process.
The software application is also able to determine whether the ladle took a break in the journey at a purging station and the temperature drop due to waiting at the purging station. Appropriate alarms are provided if the wait starts going near acceptable lower limit. This ensures that the ladle arrives at casting station with metal at acceptable temperature.
Casting
The ladle is identified using its RFID at the casting station once it arrives there. This provides input for volume of molten metal. Accessing weight data using weighing sensors on casting station, through IIoT connected to it, flow rates are determined. Combining this information with configurations of casting station’s subcomponents like tundish and casting strands, the software application is able to associate heat number to each billet. For this the application additionally leverages ability to identify billet cutter activities through the interface with existing PLC that controls the cutters.
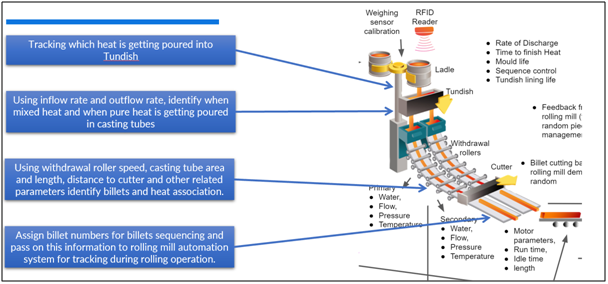
Using the sensors deployed across the conveyors transporting billets coming out of caster, the application is able to determine whether the billet has moved into rolling mill for hot rolling or has been moved to a cooling bed for future use. A barcode label creation for billet moved to cooling bed is available as option. When the cooled billet are to be reused or if billets purchased from outside are to be used, they are passed through a reheating furnace. The barcode labels provides identification os billets going through the reheating, which is a useful information for further sequencing.
Using sensors the application determines whether a billet has arrived straight from caster (hot rolling) or it has come from reheating furnace. A unique billet number is assigned to it and the appropriate heat properties are associated with this billet number. This billet number is then handed over to the Rolling Mill Automation System.
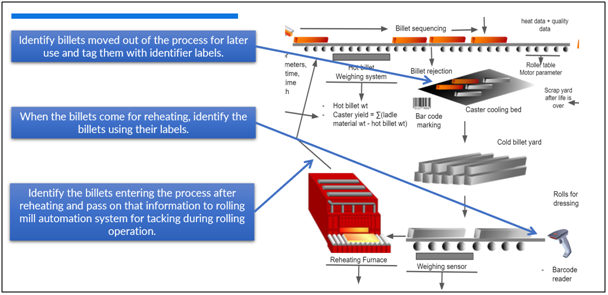
Rolling
The rolling mill automation system,in addition to its primary function, tracks the conversion of billet into bars. So when finally the bar comes off cooling bed, the Tracking application knows which billet the bar belongs to and hence knows its genealogy information like heat number it belongs to and its chemistry. The tracking application also interfaces with quality system to capture the mechanical quality test related data for the bars.
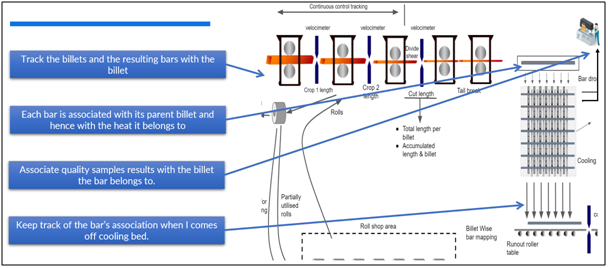
Bundling
The tracking application interfaces with PLC of bundling automation system. The application receives bundle identification number and which bars the bundle is made up of from bundling automation system. Based on this the tracking application prints a label with QR code which is attached to the bundle. Scanning the QR code gives required technical and commercial information. This QR code is useful while identifying bundle in the yard for dispatch and by the dealer / end customer to identify bars chemistry if required.
A mobile application will help dealers to scan the QR Code and instantly get chemistry details and any other details required.
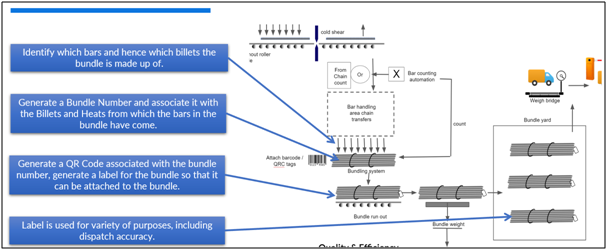
Conclusion
The current state was siloed automation in each of the areas – Melting, Casting, Rolling and Bundling. The Industry 4.0 technologies applied properly has helped to connect theses silos in such a way that it is now possible to see them as part of single process.
Before this end to end digitalisation of the manufacturing process, there was lot of people intensity involved in connecting details and information of these siloed process areas. The resulting information was prone to errors and delays. With the tracking solution deployed, it has eliminated people interventions significantly for getting information. Additionally, enrichment of the information is done in near real time to show meaningful information as the process is progressing. Ability to respond to unforeseen events has improved significantly.
It is now possible to trace the product back to its chemistry records more confidently. It is now possible to do post-mortem analysis of different dimensions related to a particular heat easily and transparently. This has paved way for getting certifications required to enhance opportunities in the markets outside India.
Outlook
The data is being collected for campaigns after campaigns and being stored on cloud. At a meaningful point in future Big Data techniques and Analytics will be applied to arrive at patterns that corelate input material, process / equipment configurations and product quality. These patterns will help arrive at, using machine learning technologies, equipment configurations required for a particular type of input material and required product quality. These configurations can be directly fed into equipment if there are capable to receive it electronically or get the setting manually done by a unskilled worker using AR based Connected Worker solutions. This will eliminate uncertainty in the expected final product for a production campaign.
About the Authors
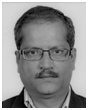
Mr. Yatin Purandare
Chairman and MD,
Vega Innovations and Technoconsultants
http://www.vegainnovations.co.in/
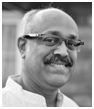
Mr. Yeshwant Satam
Executive Director,
Vega Innovations and Technoconsultants
http://www.vegainnovations.co.in/